“Let Google do the googling for you.” The debut of AI Overviews at Google’s I/O conference last May came with this promise: no more tedious searches through low-quality content, no more hunting for specific paragraphs buried in lengthy texts, and no more navigating complex Reddit threads.
This new feature integrates Google’s large language model (LLM), Gemini, which generates content in response to natural language queries. The idea is simple: ask a question, get a concise summary, and skip the hassle of searching, filtering, and finding information.
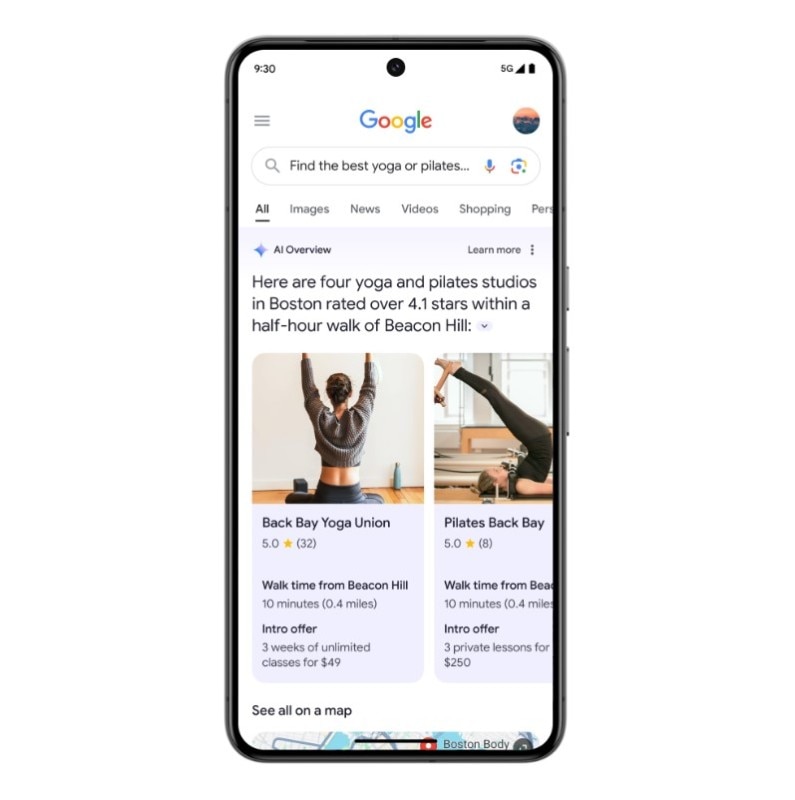
At least, that was the plan. As expected by many in the industry, things didn’t go quite as planned for the tech giant. Within days, reports started pouring in about AI Overviews making incredible mistakes. For instance, it suggested adding glue to pizza and recommended eating a small rock a day. It even incorrectly described Barack Obama’s religion as Muslim, when he is actually a Protestant Christian.
How Overviews works
To understand why a system designed to “Google” for us is suggesting health tips and endorsing theories from the US far right, we need to delve into how AI Overviews operates. So, how does it work? At its core, AI Overviews relies on a large language model (LLM). This artificial intelligence algorithm is trained on vast datasets that include extensive texts, such as the entire English-language Wikipedia, articles from major newspapers worldwide, all content from platforms like Reddit, and more.
By analyzing this vast amount of text, the LLM learns to generate text by predicting the most likely word to follow a given word. For instance, it might predict that “I’m taking the dog” would likely be followed by “for a walk.” However, it’s essential to understand that the AI doesn’t comprehend concepts like “dog” or “walk.” Instead, it makes predictions based on statistical patterns derived from the immense volume of data it has processed.
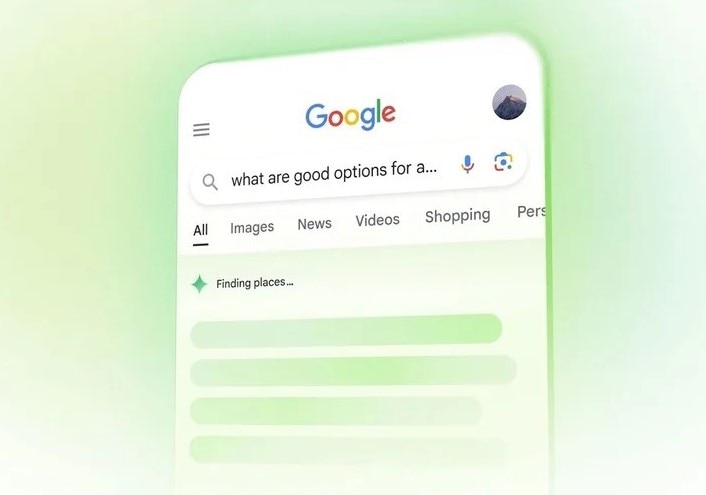
Most of the time, everything goes right, and the large language model is able to provide correct information. However, in many cases, these systems can present false or entirely fabricated information as fact. For instance, Australian politician Brian Hood, known for his anti-corruption crusades, was falsely described by ChatGPT as a “corrupt politician arrested for corruption” (information removed after legal threats from Hood). Meta’s Galactica also propagated a completely fabricated news story about some bears being sent into space, presenting it as historical fact. Furthermore, these models have been known to spread nonsense on social media, such as declaring that Hillary Clinton was President of the United States, or erroneously stating that a pound of iron weighs more than a pound of feathers – these are categorized as “hallucinations” in technical terms.
How did we get to glue on pizza?
AI Overviews works differently than a regular large language model. Specifically, Google’s system combines the capabilities of an LLM with those of a traditional search engine. In short, when we ask AI Overviews a query, the system crawls Google, identifies the sources it considers most reliable, and then reconstructs the information to produce the content we are looking for.
Within days, reports started pouring in about AI Overviews making incredible mistakes. For instance, it suggested adding glue to pizza and recommended eating a small rock a day.
It is therefore a two-step process: online research based on Google rankings and revision of the identified sources. This should make the system more “grounded” (as insiders say): more concrete, more solid, and based on quality, constantly updated information.
So how do we explain hallucinations like glue on pizza and rock diets? The problem is that the Internet is full of memes, jokes, sarcasm, misinformation, propaganda, and more. These systems, which rely on site rankings and their ability to rehash content, don’t always succeed in finding the best sources and interpreting them correctly.
For example, the news about Obama’s alleged Muslim faith was caused by an academic paper identified by AI Overviews that debunked this and other conspiracy theories. Although the paper was accurate, it had an ambiguous title: Barack Hussein Obama: America’s First Muslim President? As MIT Technology Review notes, “not only did the AI system miss the entire point of the essay, it interpreted it in the exact opposite of the intended way.”
The same thing happened with the glue and rocks: the information came from ironic threads on Reddit. The problem here is twofold: the AI’s inability to distinguish between a joke and a serious statement, and the fact that Reddit has significant relevance for Google’s search engine, which often ranks it highly. “Just because it’s relevant doesn’t mean it’s right,” sums up Chirag Shah, a lecturer specializing in online research.
Is the web too complicated for AI to understand?
Another challenge is navigating conflicting information. Imagine searching for technical details in a manual with multiple editions over the years. An AI system may struggle to identify the most current and reliable information, offering a single perspective where human searches might yield diverse viewpoints.
In short, these systems are better at appearing reliable than they actually are. The more specific the topic, the harder it is to tell if the system is wrong.
Of course, it’s possible to improve Overviews. Google is making technical updates to reduce the likelihood of errors by using human feedback (where people perform searches and evaluate the responses) and flagging sensitive terms that shouldn’t generate a response.
But the truth is these systems can never be 100 percent accurate. The more we rely on them, the greater the risk of bad information, especially on critical topics like health, finances, and politics. When we do a Google search today, we’re responsible for evaluating and using the information we find. With AI, we delegate that responsibility to a system that pretends to know everything without actually knowing anything.
So, why do companies like Google, Meta, and OpenAI continue down this path? New York University professor Gary Marcus offers an explanation. He noted that the economic returns of generative AI are modest overall. The hope of generating large revenues lies mainly in integrating language models into search engines, boosting an industry already worth about $225 billion.
The problem for information sites
AI systems aiming to “Google for us” present another problem. The goal of AI Overviews and similar tools is to create a “zero-click” search, where we don’t have to click on any website to find the information we seek. If this is the future, how will the sites that produce the information AI Overviews draws on be compensated? When AI can autonomously analyze a YouTube video to extract information, how will the creator of that video be monetized?
Until now, Google’s job has been to guide us through the vast web. Now it aims to eliminate the need for navigation. However, by removing economic incentives to produce content, AI-based search engines risk the disappearance of the material they rely on. ChatGPT, AI Overviews, and others can’t create original content; they can only remix existing web content.
As the New Yorker summarized, “Google and OpenAI seem poised to cause the erosion of the very ecosystem their tools depend on.” This is a significant issue that needs more attention.
Opening image generated by Gemini, Google'AI